Mountains and risk heuristics
I need a heuristic to be simple enough to do in my head, while doing other things like observing and engaging. Sounds trivial but isn't for a lot of people and it is great to see society being able to talk about thinks like autism and ADHD with maturity. This heuristic about decision risk, which is the chance and cost of being wrong where wrong is an available option which we would have taken, if only we'd known. The aim is to be able to see the boundaries of the risk event in question, which is something we think can/might happen and which we don't want to happen. I don't really do the upside definition of risk, which traps us in odd language like "dis-benefit".
I want to understand the risk event in several dimensions:
- how much of the risk problem set do the people responsible for controlling the risk event and the corresponding opportunity think they have solved
- the extent to which they have covered the four dimensions of ethics, uncertainty, epistemics and analytics
- how to associate an overall control state to an overall maturity state that I can kick the tyres on and be happy with
This example is in the application of governing data, but the model is application independent. The spread of numbers at the bottom is the likelihood spread and I've identified a threshold state by moving the numbers to the back end of the mountain to be climbed. This means for me to be confident a risk state is governed I'd expect to see the ethics, uncertainty and epistemics covered off. That's genuinely a lot of ground to cover and indicative as the boundary between managed and governed is fine and does shift.
I also have a positive view of regulation. All the parts in an engine are regulated, exchanging energy as efficiently as possible. A well-run gamer crew on Discord can completely outperform a non-gamer team on a similar task set because they have found a way to regulate behaviour, even though they might all be ADHD or autistic. The point being is that we seek quite a high level of coordination and exchange in our systems: we want them to be well-situated in a well-positioned network. This is to some extent the essence of business strategy.
What I didn't learn from information design I picked up from art school. Emphasising again for a heuristic to help me I need to be able to visualise it so the trips to art school were very useful (and I sold five pieces out of 21 which I think is a good cross-professional validation). I like models which push people forward and which give people the space to form and reform their understanding of the vision.
I grew up on a Wairarapa hill country farm, ended up doing a lot of hiking and bushcraft training before many years later working on risk for New Zealand Search and Rescue context and putting a risk presentation together for Alpine New Zealand. When you climb one hill, many more are revealed. The ground cuts away, the light angles change, the over-familiarity means you don't see the mountains for the hills.
In technology project work the world can be broken down into smaller pieces (but no smaller than a Planck unit) and this is all that slog and organisation needed to get to the top. But once at the top, there is nothing else but to walk down the other side and do it all over again. This describes both the passage of the mountaineer and the work schedule of the program team. Work can also turn into a nested mass of gullies, ravines, draws, ridges and folds. Some of the most chaotic geological structures to study is in Estonia where large sections are covered in glacial moraine, producing all sorts of strange spatial effects.
Why present work this way, why present a model which shows work is tiresome, long and hard. Well, aside from that being what work has been for most people, it speaks to commitment, discipline, organization and willpower. If you want to get your risks well-regulated, you have to go up and down a few mountains first. If this feels absurd, consider Albert Camus and The Myth of Sisyphus,
At the very end of his long effort measured by skyless space and time without depth, the purpose is achieved [of pushing a big rock up a hill]. Then Sisyphus watches the stone rush down in a few moments towards that lower world whence he will have to push it up again towards the summit. He goes back down to the plain.
It is during that return, that pause, that Sisyphus interests me. A face that toils so close to stones is already stone itself! I see that man going back down with a heavy measured step towards the torment of which he will never know the end. That hour like a breathing space which returns as surely as his suffering, that is the hour of consciousness. At each of those moments when he leaves the heights and gradually sinks towards the lairs of the gods, he is superior to his fate. He is stronger than his rock.
If lost in mountains, it's possible to use Bayesian methods to get out of a set of mountains or spend a lot of taxpayer's money getting lost in New Zealands unforgiving forest environment and NZSAR will come and find you. It's also possible to use a map for a different set of mountains to orient the basic mental model. But most people will use a local map, a compass, a strategy and a weather report. Interestingly many a technology driven intervention into search and rescue will stall out in heavy forest and mountains. This makes orienteering one of the single best things any human can do: the knowledge doesn't need a battery or wifi.
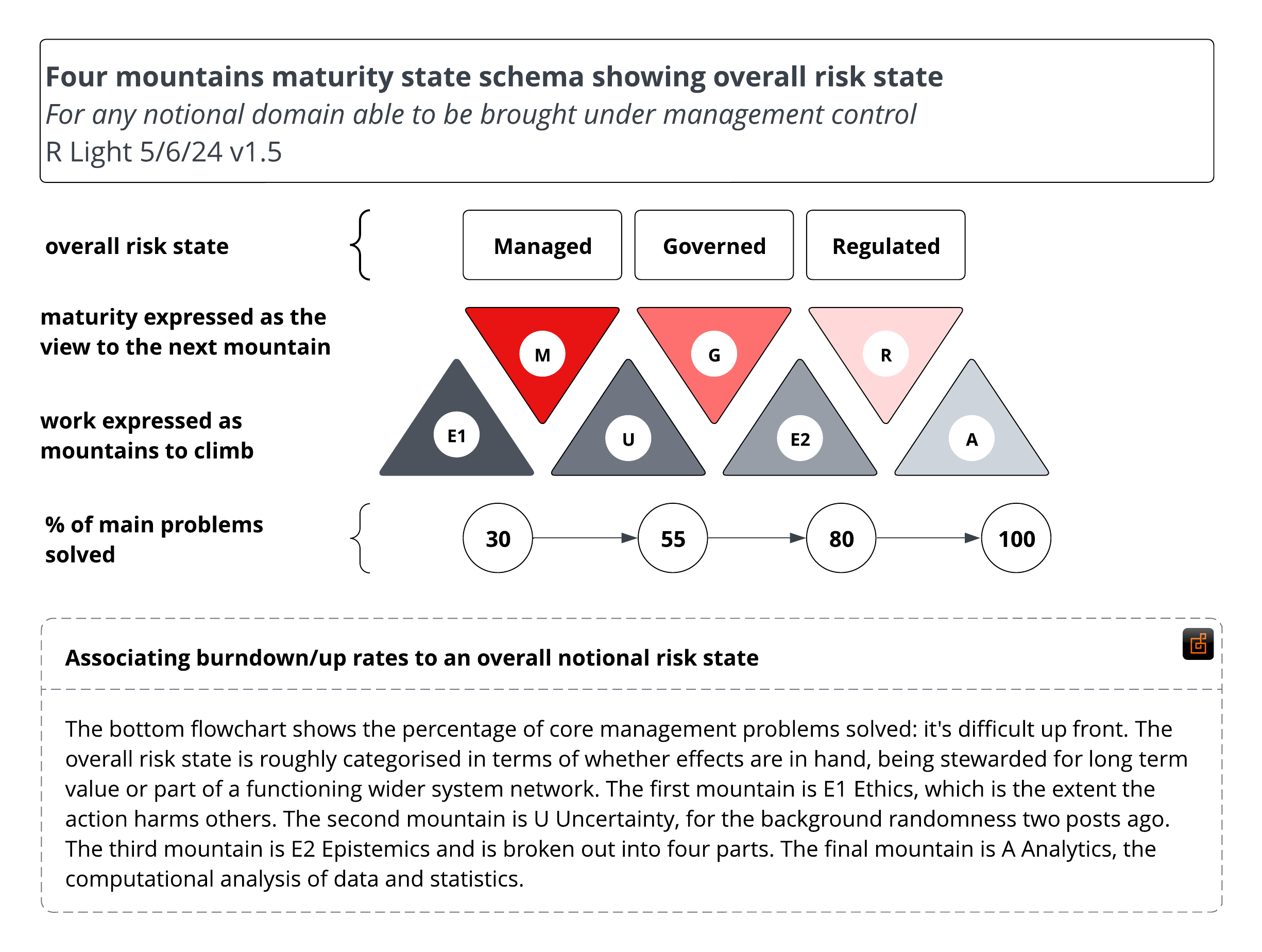
Models are normative and this one starts with ethics as the first factor so is clearly making a statement. What am I saying by defining such a thing? I'm saying that only 20% of the problems we get wrong are wrong because of our analysis. Furthermore, these analytic problems are dependent on prior epistemics, uncertainty and ethics. It is true to say these four can be shown as a circle because they do interact: I'm saying there is a hidden priority order which we can't see circular information designs. Some years ago, we jumped straight to the analytics and now we're paying for that jump with our interaction problems with the current crop of large language models
Back in the day I had a rule that every data problem masks a social problem and that still pretty much holds. In the years since I've had the great luck to work with philosophers of ethics and applied practitioners, with a host of useful explanations and constructs. There are many people much better than me at structuring these frameworks so I ended up looking for the simplest push me-pull you relationship I could find. I ended up with power-in-tension-with-trust. I used to have it as power-in-tension-with-change, but the change complement kept falling over.
Thus, the ethical risk check is about imbalances in the relationship between power and trust. Where there is a big imbalance there is likely to be a large pile of consequent problems that need working through, in addition to the uncertainty, epistemics and analysis which we already have to do. Enabling a channel for truth to speak to power is imho an important part of dealing with the messy issues, before we find ourselves in a cascade of avoidance decisions because we dodged the hard one up front. In risk we aren't in the business of not knowing how messy things actually are. Most other people want to diminish and avoid but we like to know the worst because, quite probably, the risk pro knows of a worse one.
Once we're on a solid-ish basis with morals, ethics, values and all that fun stuff, we approach the matter of uncertainty. This can be understood using structured language centred around likelihoods, distinguishing uncertainty from risk and recognising the background randomness of a room full of copiers is different in a floor full of clinicians than it is a building full of travel agents. My sense is that for most people uncertainty and its attendant terms very much mean DANGER. It shouldn't be but it is and many a person with a sharp eye on the 100% probability distribution has made a career from this. I've covered uncertainty all through this blog so will leave uncertainty for now.
This also means however that I'm comfortable to some extent that a risk is being managed: the team understands the impact of what they do on others, and they have some notional distinction between likelihoods, randomness, uncertainty and risk. The risk management professional who moves between teams do see the differences between teams and very often these two are the absolute basics.
Which means now we're into the epistemics, which I ground by distinguishing the notion of epistemic rationality (what is true) and instrumental rationality (what to do). Another way into this is recognising that while most people will recognize they think, few will then recognize that most people think with ideas and then even less will recognize we mainly take the ideas we think with from our environment and do so mostly unconsciously. We are mere sieves of ourselves so to speak.
This area has taken me since about 2016 to stack and restack all the inputs until common points resolved and we end up with a reduction of requisite complexity. Not that there is always going to be some requisite complexity we need to leave in our system. I show that the epistemics mountain E2 is large because it has 45% of the risk surface to be managed/problems to be solved. But a nested model can't stay inexplicable for long and should be placed close to where its nested.
Which means an additional expression of the four mountains. However, that's no fun so here is the model I used to get to this point, which I called the grasshopper.
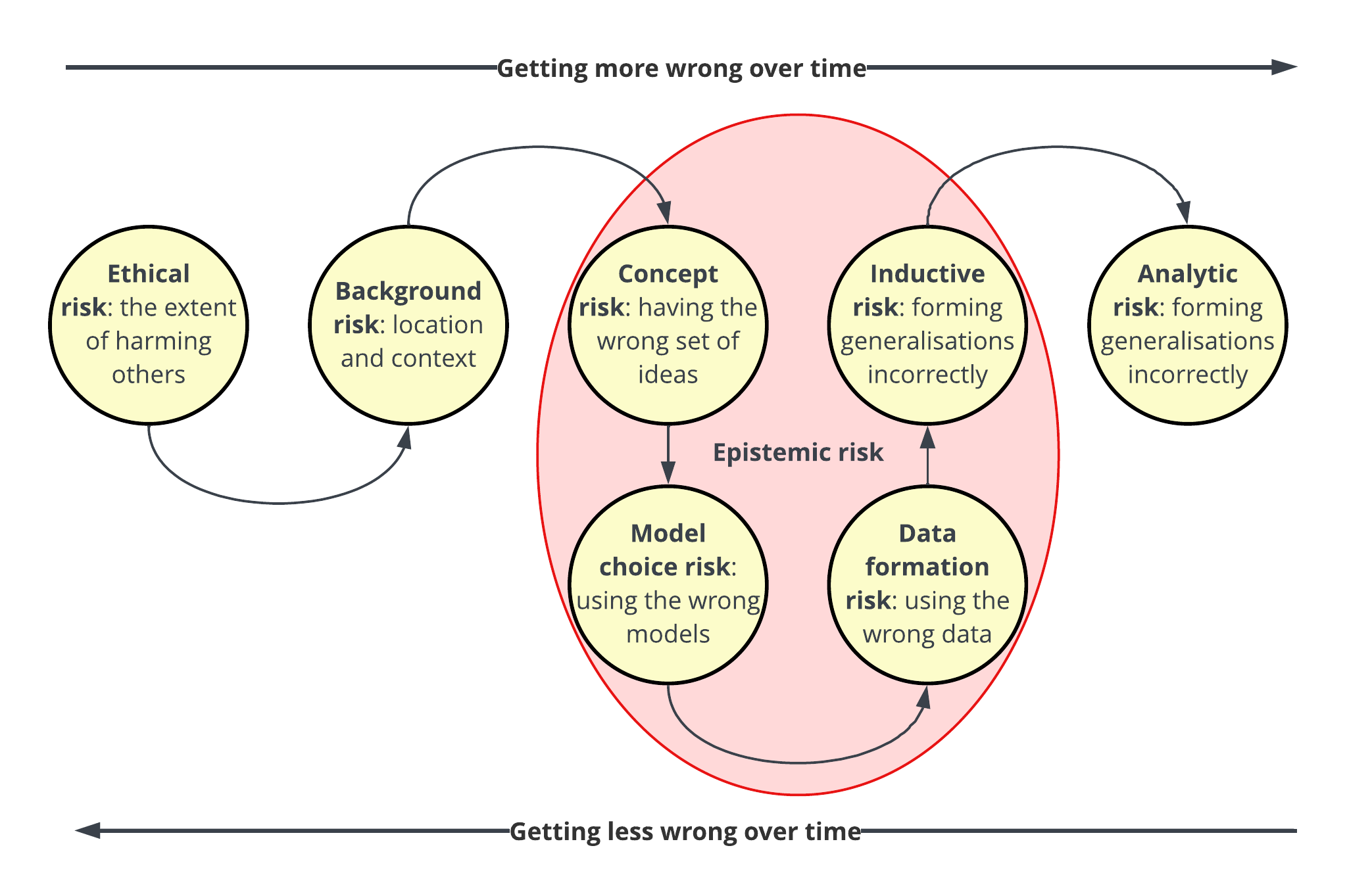
The useful thing about covering off the interplay of the four epistemic risks is we have a good claim to having the problematic risk event in question into a governed state. Risk management pros will recognise this is also the core of the model risk management body of work. It took a while associating that overall methodology as being able to control all four epistemic risks but that's what testing is for. There's still work to do with the back end of the four epistemic risks, but the hard work is done and the climb down now is all about getting all the evidence and facts lined up.
There will be different industry requirements around regulation. The overall sense I get is that the increased regularisation and conformance of our AI society will help identify when regulation is really, really useful and when it's just a dog whistle. That regulated state requires a lot of analysis and here is the modern kicker: if we haven't actually solved for all those previous stages, this is where a large language model has already eaten our lunch because they work in machine time and we're still diurnal.
I think the progression is about right, the state identification is the same, colours and fade outs are done; the number spread and circle location I'm still working on. To all my colleagues who became friends and asked me how I think into the problem space, this is it and this is for you.