Don't use shortcuts taking the test of experience
A theory of change helps apply the test of experience to our ideas and in so doing enables them to be deemed empirical.
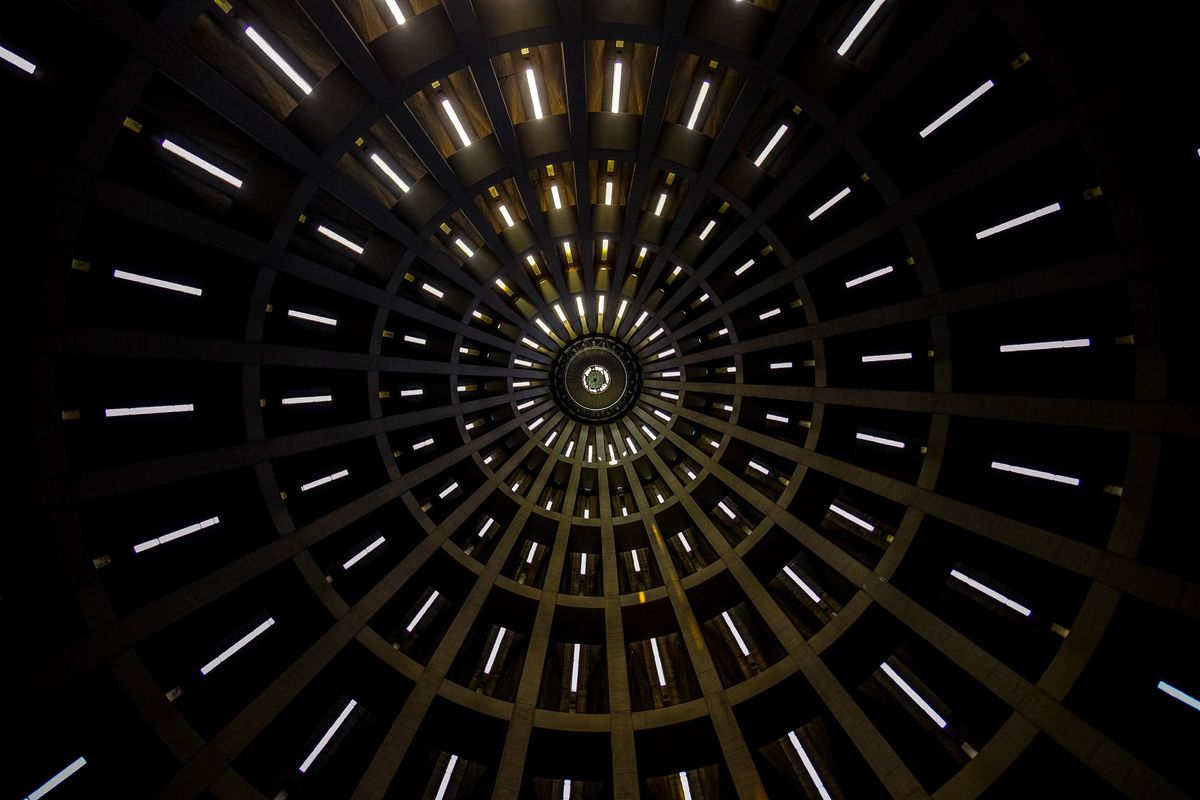
In my previous post I wrote about how we should locate our lean start-up and agile methodologies within a theory of change. Over the next few posts I'm going into more detail about why I think this is important.
First though, a little detour. Many folk (me included) are a bit lazy when it comes to doing the hard yards. We take shortcuts in the hope that we can get around difficult requirements.
Many times these shortcuts do the job. The rest of the time, they only seem to the do the job. Until it becomes obvious that they didn't. After a while taking shortcuts, become a habit and then are normalised, after which they are often expressed as 'saving' time (which can't be done, but never mind).
I resisted working to the rigour of a theory of change and took time saving shortcuts for quite a while. I build change capability and people are averse to the loss that comes with change. Which means turning up with a 'solution' that makes life more difficult for something that doesn't seem broken is a hard sell indeed.
However, after a while I noticed two things: there are a heck of a lot of shortcuts available to people and many of those shortcuts lead to future quality problems. Because successful change capability work is based to a significant degree on mitigating, reducing or eliminating future quality problems, I had to stop taking time saving shortcuts that took me to the wrong outcomes.
I also work in data governance, which in the current environment (among many other things) is focused on reducing future quality problems with machine learning. So I now needed a way to sort good shortcuts from the bad. I found that once I adopted theory of change methodology and stopped taking my own shortcuts, I could see the well worn ones others were taking.
What I found was a veritable feast of future quality problems. I also noticed a decreasing time cushion before these quality problems are realised. We can see these technique-based quality problems emerge as professionals around the world apply different doctrines to the same set of problems (we're not as heterogeneous as we'd like to think) and come up with wildly divergent policy responses.
In a surprisingly short space of time, these technique-based future quality problems get very large indeed. At this point, it is wise to note that the mitigations for these future quality problems are mostly found within or near the disciplines needed to work through a theory of change.
So we may as well just do it already.
OK, what's a theory of change? It's,
"a theory of how and why an initiative works, which can be empirically tested by measuring indicators for every expected step on the hypothesized causal pathway to impact"
(De Silva, M.J., Breuer, E., Lee, L. et al. Theory of Change: a theory-driven approach to enhance the Medical Research Council's framework for complex interventions. Trials15, 267 (2014). https://doi.org/10.1186/1745-6215-15-267).
When we come across words like 'empirical' and 'hypothesized causal pathway', we can see why taking shortcuts seem so appealing. We know this is going to be hard just by looking at the sentence. Unless we have a background that works within the scientific method. And even then we are probably going to look for a shortcut for a better explanation. Because that's what humans do.
The important thing here is that a theory of change will help us both identify a bad shortcut for what it is and help turn a not-so-bad shortcut into an ok or good one. It's the last option I like the most. It means everyone can obtain a performance improvement because we're all a bundle of shortcuts and heuristics anyway.
Back to decomposing the definition. We want to understand the important bits that we avoid and end up regretting, either through bad shortcuts or path dependence. The core of what can't be avoided is (i) 'empirically tested by measuring indicators' and (ii) the 'hypothesized causal pathway to impact'.
We need the first element to understand how far we've gone (and how far there is to go between our current position and an imperfectly known target future state) and the second to explain that what we're doing will make, is making and has made a difference. So, kind of important stuff here.
One useful thing to get out of the way at this point is the word 'test'. Reducing ambiguity of terms as much as possible is a useful habit. After tidying up the areas where people 'agree vigorously', we will be left with residual ambiguity. This will probably be associated to difference in usage between professional groups.
The task here is to identify the extent to which ambiguity of terms agree and disagree.The reason for this is some "testing" will end up with the 'empirical' tag and others will end up with the 'everything else' tag. This becomes a data governance issue because the trajectory of our work includes third party oversight of machine learning.
It will eventually become a case of empirical in and non-empirical out for high grade machine learning: how a data set is created is critical to how seriously it can be taken by a wider spread of people at a later date. Evidence is politically situated, and wide acceptance is akin to progress.
One of the big issues in data governance is the (widely attested) cultural, racial and gender bias in historical data sets. Which is a big part of 'big' data. This can be (largely) mitigated by the application of statistical control, information management, and data governance . Hence why empiricism is important.
So we want to understand what people mean when they say 'test'. Sometimes test means going to a colleague and asking them for their opinion, peer review or written input. Sometimes we test by running a sufficiently large number of people through a structured instrument. Sometimes we test by running software and looking for problems in code.
Sometimes we test in the context of seeking to disprove a hypothesis. Sometimes we test in the context of seeking to identify a sustainable, value-producing product and service. Sometimes we test to identify the degree of caution we should observe in order to move work successfully through our political environment.
That's a fair chunk of ambiguity and helps us understand how easy it is to end up with the 'everything else' tag. The good news is we can find the flexibility and wiggle-room we need within the scientific method (from which theory of change methodology emerged):
"No human investigation can be termed true science if it is not capable of mathematical demonstration. If you say that the sciences which begin and end in the mind [i.e. Aristotelian natural philosophy] are true, that is not to be conceded, but is denied for many reasons, and chiefly the fact that the test of experience is absent from these exercises of the mind, and without it nothing can be certain" Leonardo da Vinci
Don't get too bothered about the "capable of mathematical demonstration" bit. We do this every (or every other) day, its just that we may not realise it. The important part is we have to get ideas out of our mind and subject them to the test of experience. Note at this point there is a minimum threshold for this testing. And testing in the political sense falls below that threshold.
A quick check of that most excellent validated benchmark for reducing ambiguity in language (the Oxford Dictionary) tells us that the adjective 'empirical' means "based on, concerned with, or verifiable by observation or experience rather than theory or pure logic." This is what a theory of change does for our work. It takes ideas out of our minds and gives them a chance to get the 'empirical' tag.
Many of the shortcuts we take rob our work of their empirical potential. This is the source of a lot of future data governance quality problems. If we can't get our ideas into some empirical form, then at some point in the future someone is going to downgrade the quality of our work.
Which is something I assume most professionals want to avoid. Maybe I'm wrong, but I don't expect to see 'expert analysis-by-Sharpie' on successful resumes.
A theory of change helps apply the test of experience to our ideas and in so doing enables them to be deemed empirical. It is in this way we help sort fact from opinion and move the world to a better place.
Photo by Francesco Ungaro from Pexels